基于RFECV特征选择和随机森林预测模型的应用与优化
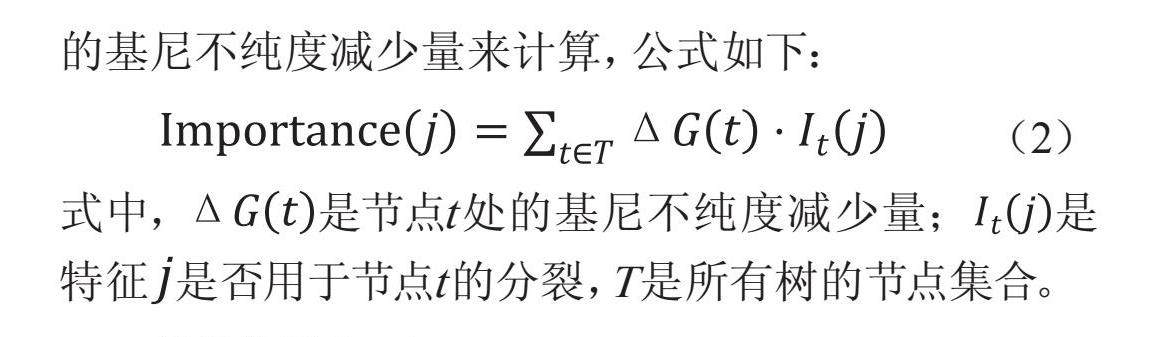
打开文本图片集
摘要:该文基于随机森林预测模型,提出RFECV特征选择方法:首先对特征变量进行独热编码,再利用RFECV内置的交叉验证评估各特征子集性能,以确定最佳特征数量,并递归消除低重要性特征。实验表明,该方法在随机森林上训练与预测更快,均方误差更低,特征提取准确率高。
关键词:随机森林预测模型;独热编码;递归特征消除;交叉验证
doi:10.3969/J.ISSN.1672-7274.2024.09.039
中图分类号:TP 391 文献标志码:B 文章编码:1672-7274(2024)09-0-03
Feature Selection Based on RFECV and Application and Optimization of Random Forest Prediction Model
SUN Jing
(School of Computer Science and Technology, Taiyuan University of Science and Technology, Taiyuan 030000, China)
Abstract: Based on the random forest prediction model, this paper proposes the RFECV feature selection method: firstly, the feature variables are encoded with one-hot encoding, and then the built-in cross-validation of RFECV is used to evaluate the performance of each feature subset to determine the optimal number of features, and recursively eliminate low-importance features. Experiments show that this method achieves faster training and prediction on the random forest, lower mean squared error, and high accuracy in feature extraction.
Keywords: random forest prediction model; one-hot encoding; recursive feature elimination; cross-validation
0 引言
在数据量高速增长的今天,与数据对象相关的其他特征数据越来越多,在分析的过程中,不可避免要对这些特征数据的影响力进行计算并判断,从而更好地理解数据对象,服务于后续流程。(剩余4688字)