基于改进YOLOv4的实时目标检测方法研究
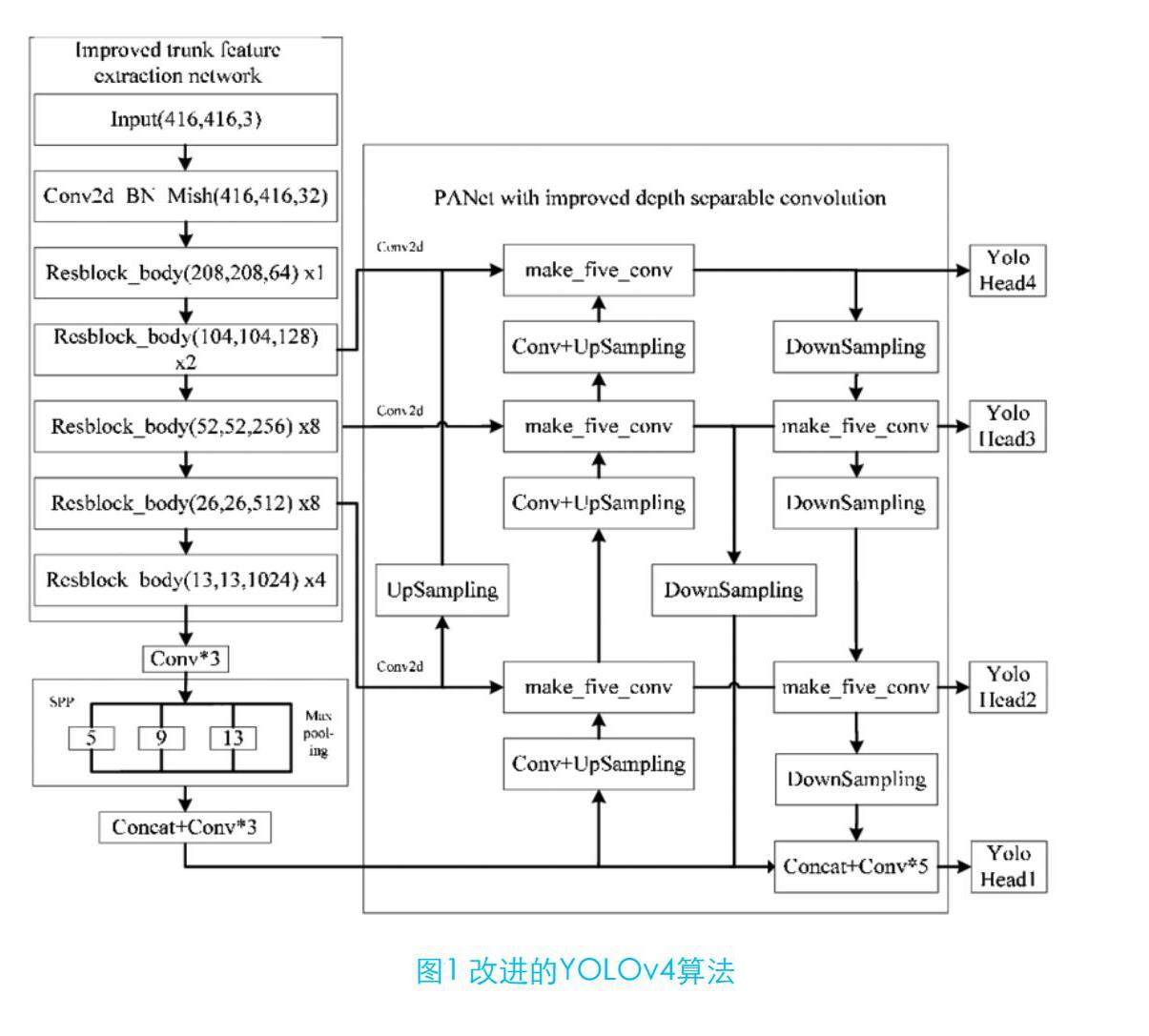
打开文本图片集
摘要:为提升实时目标检测的准确性和稳健性,该文采用增强特征融合技术、网络架构技术、损失函数技术等对YOLOv4算法进行优化。结果表明,改良后的YOLOv4算法在多变环境下对小型目标检测表现出色,展现了其实用性和稳定性,为广泛应用奠定了坚实基础。
关键词:实时目标检测;YOLOv4;特征融合;GIoU损失函数
doi:10.3969/J.ISSN.1672-7274.2024.09.006
中图分类号:TP 31 文献标志码:B 文章编码:1672-7274(2024)09-00-04
Research on Real-time Object Detection Method Based on Improved YOLOv4
LU Jianheng
(College of Artificial Intelligence, Guangzhou Huashang College, Guangzhou 511300, China)
Abstract: To enhance the accuracy and robustness of real-time object detection, this paper optimizes the YOLOv4 algorithm by employing enhanced feature fusion technology, network architecture technology, loss function technology, and other strategies. The results demonstrate that the improved YOLOv4 algorithm exhibits excellent performance in detecting small objects in diverse environments, showcasing its practicality and stability, and laying a solid foundation for its widespread application.
Keywords: real-time object detection; YOLOv4; feature fusion; GIoU loss function
0 引言
在人工智能与视觉科技领域,实时目标检测技术日益凸显其重要性,广泛应用于自动驾驶、视频监控、无人机导航等多个领域。(剩余4785字)