基于机器学习的女性压力性尿失禁发病风险预测模型建立及效能评价
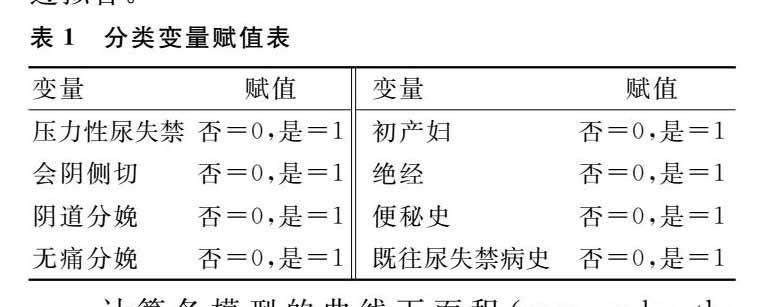
打开文本图片集
ABSTRACT:ObjectiveTo construct prediction models of female stress urinary incontinence (SUI), and evaluate the efficacy of each model, so as to provide reference for the early diagnosis of SUI. MethodsFemale SUI patients treated in our hospital during Oct. 2019 and Oct. 2023 and healthy women undergoing physical examination during the same period were involved. Women 42 days after delivery were included in the postpartum group (n=611), and perimenopausal and postmenopausal women were included in the non-postpartum group (n=409). The number of random seeds was set and the participants were divided into the training and verification sets in a ratio of 7∶3. Relevant clinical data were collected, and meaningful variables were screened using single factor and Lasso regression, which were then incorporated into the K-nearestneighbor method (KNN), support vector machine (SVM),decision tree (DT) andrandom forest (RF) algorithms. The sensitivity, specificity, accuracy and area under the receiver operating characteristic curve (AUC) of the models were calculated to screen out the optimal model. ResultsThere were 352 SUI patients (57.6%) in the postpartum group. According to single factor and Lasso regression, significant variables included age, body mass index (BMI), maximum rapid muscle stage, parity, bladder neck mobility (BND), urethral rotation angle (URA), lateral perineal incision, past incontinence, and constipation. In the verification set, the AUC of KNN,SVM,DT and RF models were 0.881,0.878,0.750 and 0.905,respectively; the AUC, accuracy, F1 index and Kappa value of RF model were the largest. In the non-postpartum group, there were 260 SUI patients, accounting for 63.6%. The significant variables were age,BMI, maximum value and recovery time of fast muscle stage, mean value of slow muscle stage, post-resting stage variability, vaginal delivery, past incontinence, and constipation. In the verification set, the AUC of KNN,SVM,DT and RF models were 0.819,0.805,0.603 and 0.830, respectively; the AUC, accuracy, Kappa value of the RF model were the largest. ConclusionThis study successfully established 4 prediction models for the incidence of SUI in women at 42 days postpartum, perimenopausal and postmenopausal women based on machine learning. Among them, the model adopting the RF algorithm had the best prediction efficiency.
摘要:目的运用K最近邻法(KNN)、支持向量机(SVM)、决策树(DT)及随机森林(RF)构建女性压力性尿失禁(SUI)发病的预测模型,并评估各模型效能,为SUI的早期诊断提供参考。(剩余11383字)