基于 SVD 的协同过滤电影推荐算法
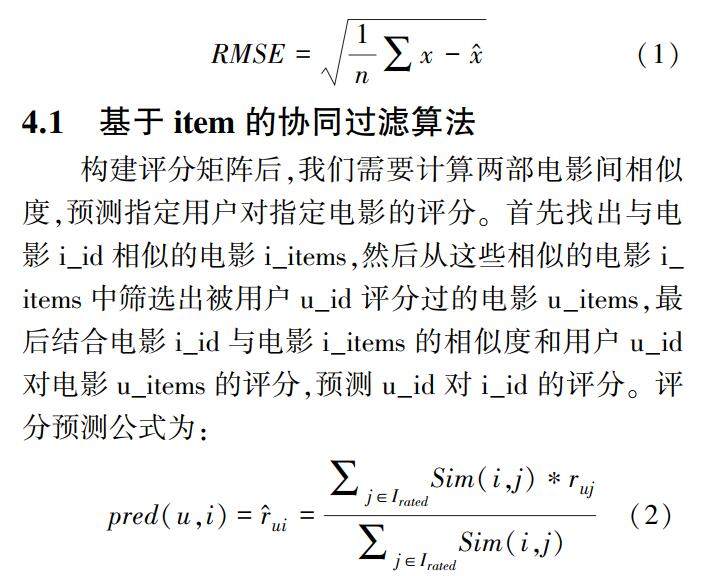
打开文本图片集
摘要:信息过载的问题愈发严重在大数据时代针对不同用户提高电影推荐系统的推荐性能一直存在巨大的挑战为了有效地解决信息过载和用户体验满意度低的问题需要选择合适的个性化推荐算法文章概述了主流的机器学习推荐算法并通过实验比较分析了各算法的优缺点针对推荐算法普遍存在的冷启动和数据稀疏性问题提出了相应的解决方案
关键词:电影推荐;协同过滤(CF);冷启动;奇异值分解(SVD)
中图法分类号:TP391文献标识码:A
Collaborative filtering movie recommendationalgorithm based on SVD
SONG Longsheng',WANG Jialel,NI Shengqiao1.2
(1.College of Information Science and Technology,Tibet University,Lhasa 850000,China:
2.College of Computer Science,Sichuan University,Chengdu 610065,China)
Abstract:Nowadays, the problem of information overload is becoming more and more serious. In the sea of big data, it has been a huge challenge to improve the recommendation performance of moie recommendation system for different users. In order to effectively solve the problems of information overload and low user experience satisfaction, it is necessary to choose an appropriate personalized recommendation algorithm. In this paper, the mainstream machine learning recommendation algorithms are summarized, and the advantages and disadvantages of each algorithm are compared and analyzed through experiments. Finally, corresponding solutions are proposed to solve the common problems of cold start and data sparsity in recommendation algorithms.
Key words: film recommendation, collaborative filtering(CF), cold start, singular value decomposition(SVD)
1 引言
近年来,随着互联网的飞速发展,影视产业的数量和种类激增且发展迅猛,“电影过载”的问题愈发严重,导致用户寻找自己喜欢的电影需要浪费很多时间。(剩余3815字)